3M™ Intelligent Control Inhaler
Enabling better breathing.
A combination of problems is to blame for rising healthcare costs and increased exacerbations in people with chronic respiratory diseases. With that knowledge, the 3M drug delivery team set our sights on revolutionizing the device + treatment landscape for those living with COPD and asthma.

600 million people
are affected, globally, by COPD or asthma, while COPD is the 3rd leading cause of death worldwide1. The combined cost for COPD and asthma is $80 billion in direct healthcare expenditures in the US alone2,3.
The problems
Low adherence
Adherence for people with pulmonary diseases is lower than many other chronic conditions4. 60% of COPD patients and up to 70% of asthma patients do not adhere to their prescribed therapy5,6.
Poor device usability
With over 14 inhaler form factors in the US, 94% of Dry Powder Inhaler (DPI) users and 76% of Metered Dose Inhaler (MDI) users make mistakes7. When an inhaler is used improperly, as little as 7% of the medication makes it to the lungs8.
Lack of knowledge
Without access to relevant information, it’s difficult to recall all the recommended trigger-specific and disease-management activities.
Environmental impacts
Respiratory difficulties are caused by many environmental triggers that are hard to detect and isolate. In addition, these ecological impacts vary from person to person and day to day.
Low resilience
Making lifestyle changes is always easier said than done. Even with the right attitude, bad habits are hard to break without consistent support.
Poor communication
It’s challenging for doctors to assess how well a treatment works when patients struggle to describe or remember their experiences.
How might we help people living with COPD and asthma reduce their symptoms, their need to use a rescue inhaler, and lower their risk for hospitalization and emergency room visits?
The inhaler
The 3M™ Intelligent Control Inhaler is a breath-actuated metered-dose inhaler developed by 3M scientists using extensive laboratory and in-vitro experimentation, modeling work with respiratory consultants, and clinical research. It has all the bells and whistles, including Bluetooth connectivity and various sensors to help improve technique during use.
Research
My team conducted 12 IDIs with patients, healthcare providers, and payers. The research highlighted the need for effective, easy-to-use, and affordable devices that empower patients, improve treatment compliance, and facilitate cost savings across the healthcare system. These were our four key takeaways:
1. It’s hard to measure change
Respiratory disease is a “non-numeric disease,” meaning it is difficult to measure changes in symptoms, disease progression, and adherence.
2. Anxiety and depression are prevalent
Patients with co-morbidities and chronic degenerative diseases like COPD experience substantial social and psychological impacts.
3. Cost and access are barriers to treatment
Healthcare providers don’t prescribe what is unavailable to their patients, even when they believe the treatments are valuable.
4. One-size-fits-all interventions
Population health programs look only at historical, clinical data for risk stratification and involve costly, labor-intensive, one-size-fits-all interventions.
N=100 study
In 2018, 3M funded a study involving 100 people with asthma to determine if artificial intelligence could predict exacerbations for individuals based on rescue and maintenance inhaler use, data inputs from a breathing sensor, weather, air quality, activity, and other self-assessment inputs. Conclusions from the n=100 study helped us understand what data would be most essential to capture.
-
Weather and pollution
Weather and pollution data increase the accuracy of any model to predict asthma symptoms.
-
24-hour predictions
24-hour predictions using 3-7 days of breathing sensor, inhaler, and self-assessment data can be as accurate as detection.
-
Individual variations
One prediction model, based on averages, is not effective when applied to individuals, because the differences in asthma response is too large.
-
Inactivity is useful
Inactivity, as an asthma symptom, could contribute toward any asthma predictor.
-
Condition severity
Models were more successful at prediction accuracies when applied to groups with similar condition severity.
-
Aggregated models
Prediction accuracies improve if independently trained models are aggregated or combined.
Competitor analysis
I scoured the market for apps utilizing connected devices to measure or track different kinds of disease progression, not limiting myself to respiratory ailments. When it came to defining and prioritizing features for our app, I narrowed my view to respiratory disease management. This space includes inhalers, inhaler bolt-ons, peak flow meters, spirometers, and breathing sensors.
Engagement
The success of our app and its algorithms would depend on the active participation of users. My colleague created an initial outline of an engagement model which logically stratifies individuals based on their level of engagement. A user’s stratification would determine the channels for communication.
The problem of adherence and user behavior
The likeliest cause of adherence failure occurs when people cannot develop a strict pattern of behavior for medication use.
Reminders alone do not solve this problem as they are only a single event, are easily dismissed, and do not remove the barriers that impede people's ability to develop habit-forming processes.
(Inter)Actions promote habit-forming practices
By providing several varied interactions, and touchpoints, we create new opportunities for users to engage with our total experience.
Interactions increase the likelihood that people will recall their inhaler, leading to more opportunities to use it and increased habit-forming behaviors that drive adherence.
Content
Creating meaningful and engaging content was crucial to our overall design strategy. I hypothesized that providing educational content based on conditions relevant to the individual would improve adherence and minimize situations that may increase the likelihood of exacerbations. Our content delivery system would use a multitude of touchpoints, and tailored content would train users to manage their unique problems.
Daily insights
Daily insights are delivered once a day. Initially, the system would provide content using a decision tree model to ensure it remains fresh and relevant based on user inputs, stratification models, and time. A machine learning algorithm would eventually provide daily insights as more data is captured.
Live insights
Live insights are contextual, real-time feedback. Outside of the user’s preferences, there is no limit to how many can be received in a day. They are triggered by logic rules that require specific conditions to be met. Initial insights were developed with example content to provide a template for how live insights could be leveraged to provide value and increase user engagement with the inhaler and the app.
Value proposition
I developed our team’s “flag in the sand” to align stakeholders. Our app would use data collection and machine learning to expose triggers and help mitigate their effects, empowering people to take control of their respiratory health.
-
Track
Track your maintenance use, technique, rescue use, symptoms, and triggers by time and location.
-
Discover
Uncover which triggers affect you and which inhaler techniques need improvement through easy-to-read data visualizations.
-
Understand
Understand your breathing experience based on your unique triggers in your location, your inhaler use, and your symptoms.
-
Be empowered
Take control of your health with help from meaningful insights delivered in moments that matter.
Architecture
I designed our site map using Miro. The primary pages include:
Today – “Today” is the application’s landing page giving users real-time, high-level summaries of the information that matters most.
Log – The “Log” shows a complete history of inhaler use, technique, and self-reported events.
Add event – Adding an event allows users to track rescue use, symptoms, and potential triggers.
Trends – “Trends” uses data visualizations to help users understand maintenance use impacts, their most common triggers, and more.
Insights – The “Insights” page keeps a history of all “Daily insights” and “Live insights.”
Wireframes and flows
My work concentrated on 14 features and 98 user stories. Together, our cross-functional team defined user stories and acceptance criteria. Following the documentation, I designed wireframes and user flows and iterated upon them based on feedback from the group.
User testing
My team conducted research to validate app features and flows using quantitative user testing and qualitative in-person sessions in Louisville, KY. A few insights:
On-boarding should be as quick as possible so that users can get to the app's contents, allowing them to receive additional context before opting into features.
Two-step confirmation of iOS permissions resulted in high opt-in.
Users believed that technique notifications would help proficiency, so long as they could be delivered before inhaler use to ensure remediation is top-of-mind.
Dose information, such as counts and reminders, was deemed highly valuable and should be prominently displayed.
Capturing inhaler habits alongside self-assessments and environmental conditions was highly valuable, giving users ways to track their condition and share that information with their doctors.
Final designs
I designed our application utilizing primitives and components from the 3M Nucleus Design System. 3M stakeholders and potential pharma partners praised the overall design and strategy, which focused on the individual, not the inhaler.

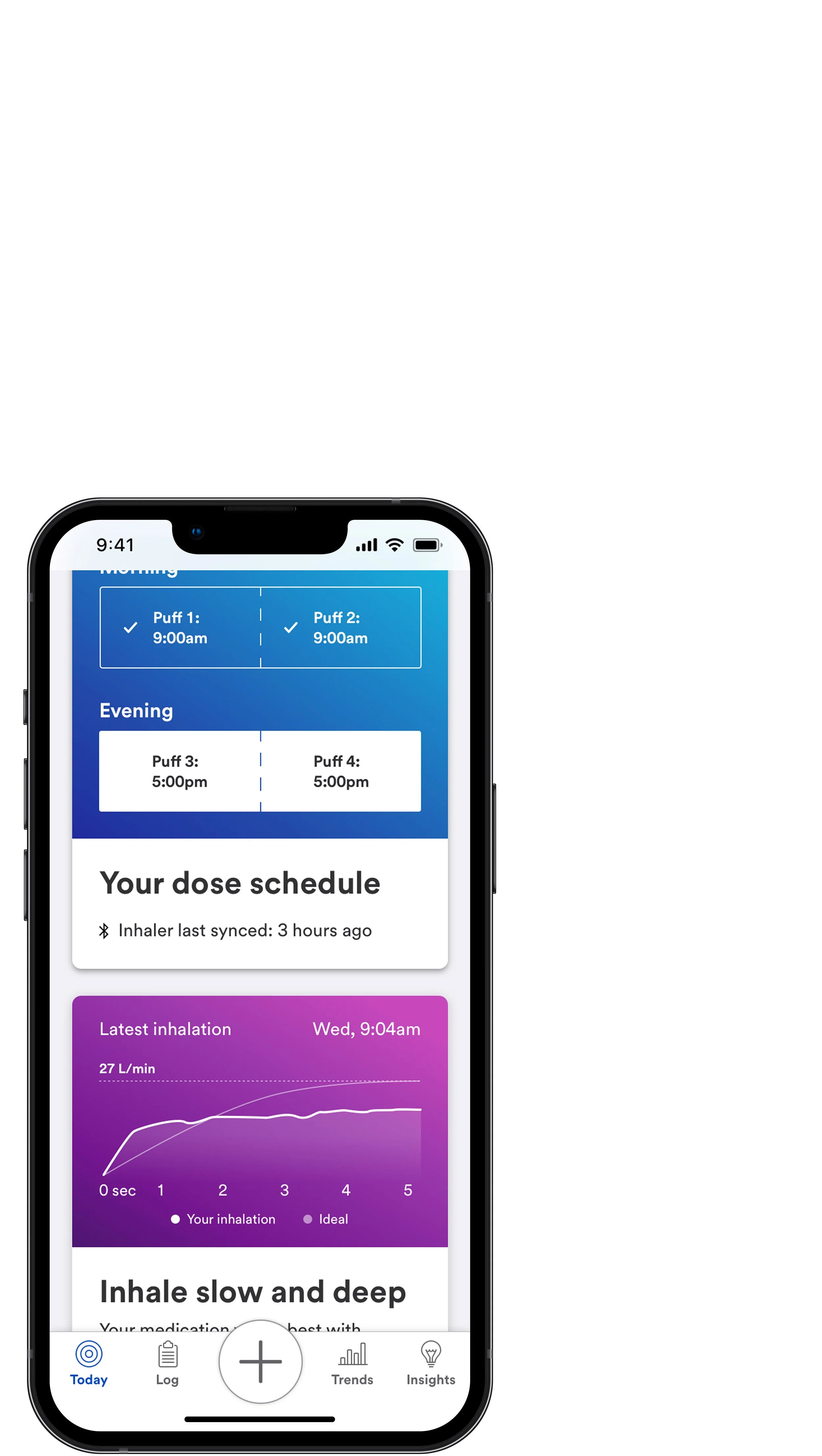
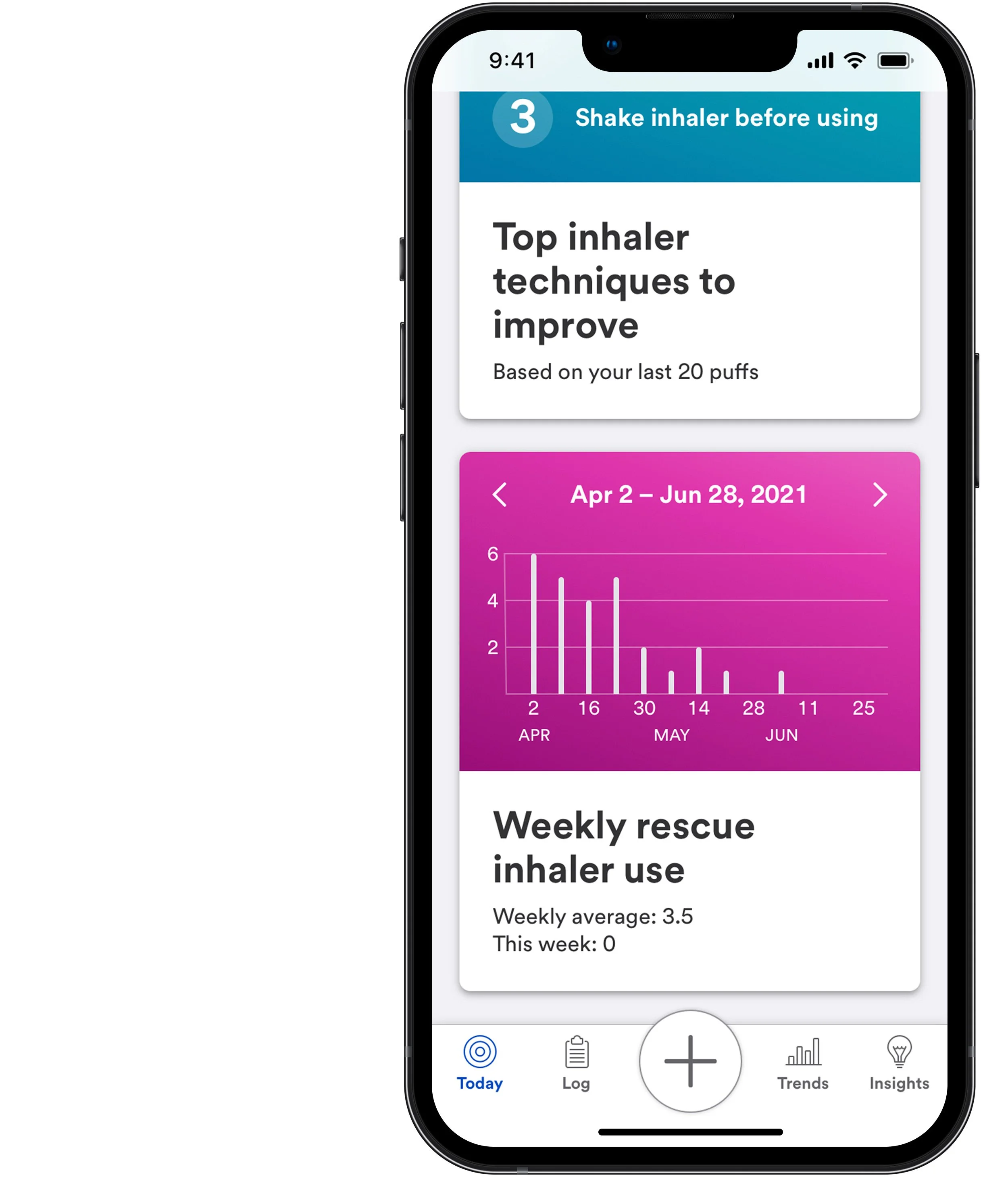

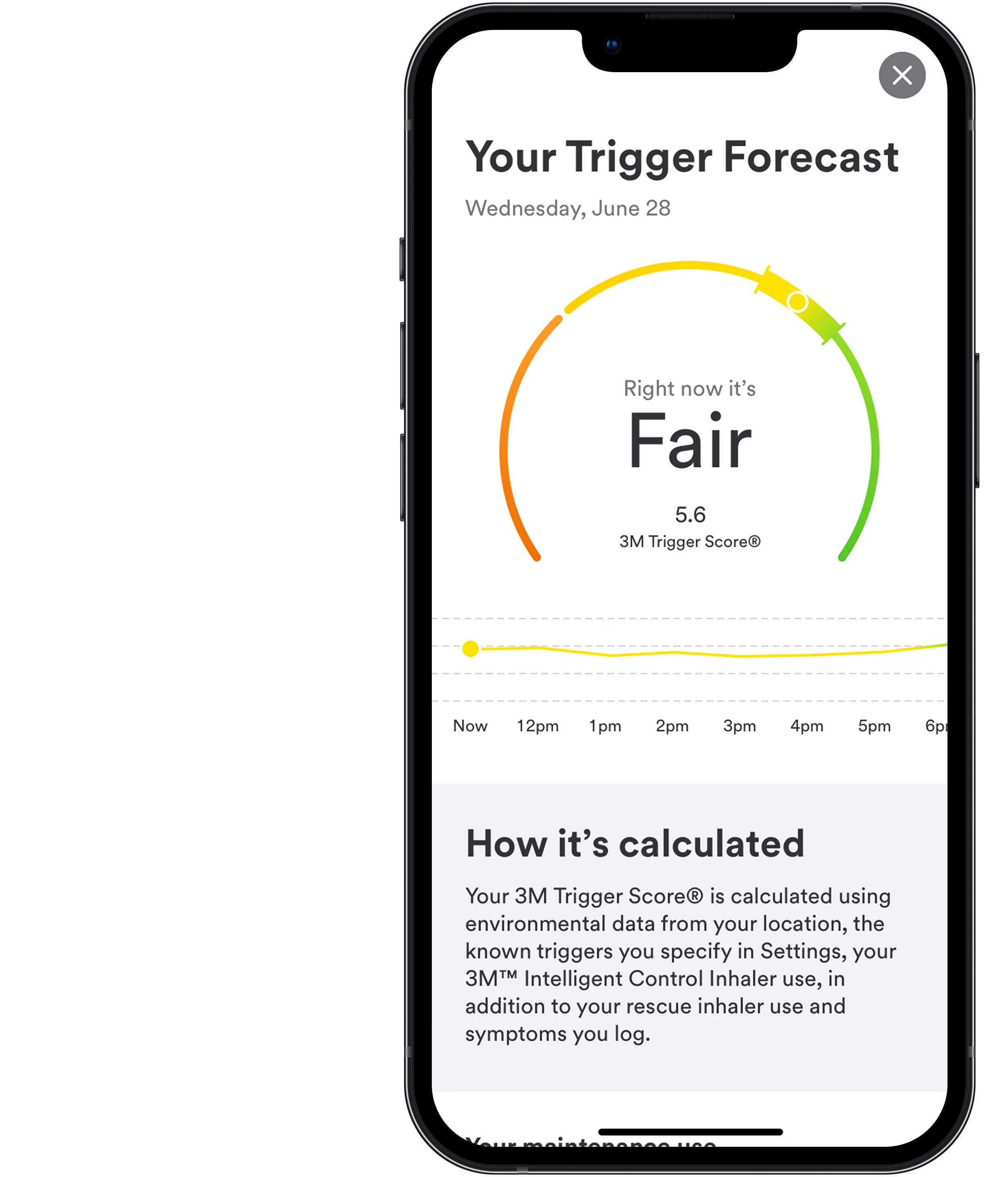
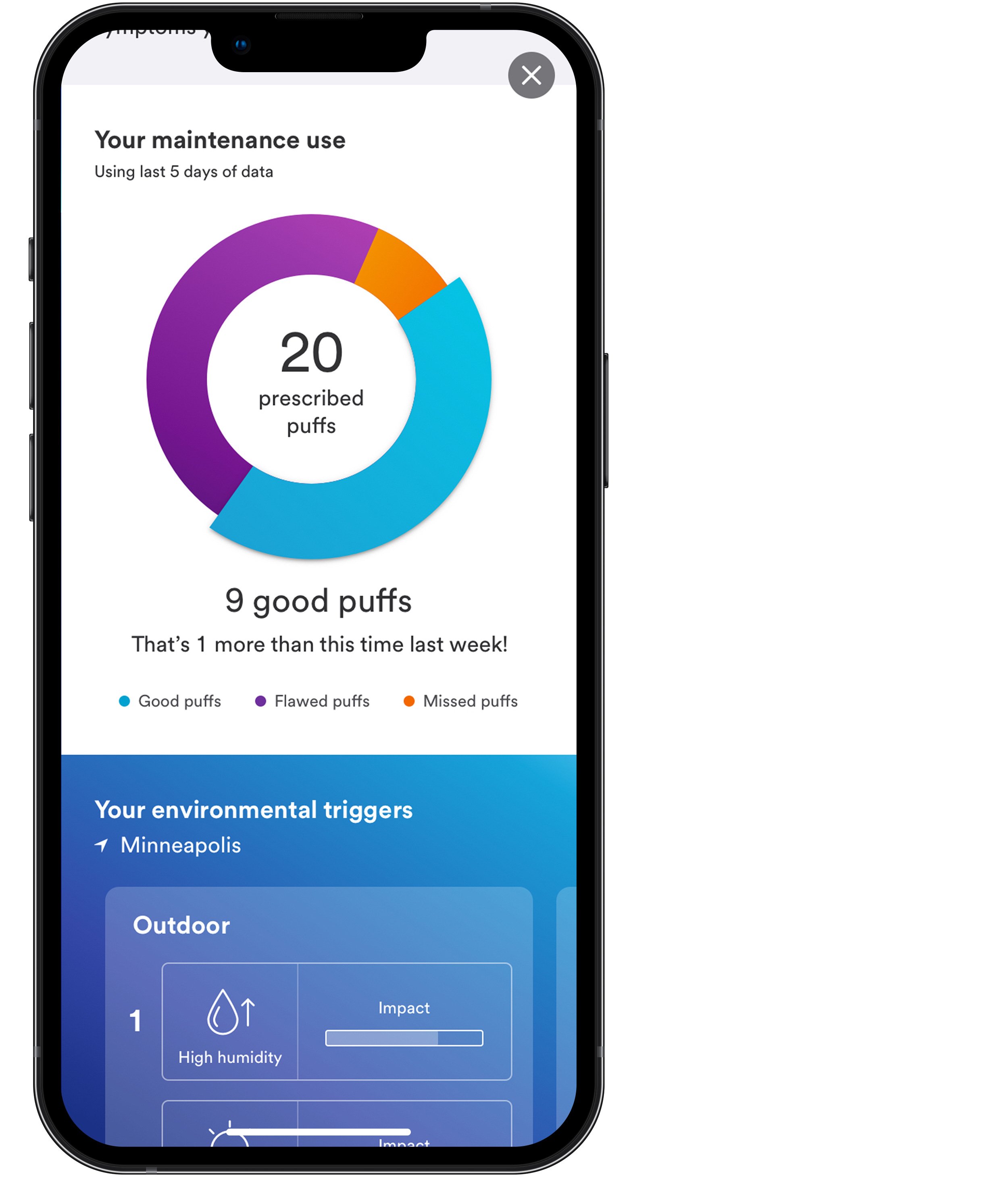
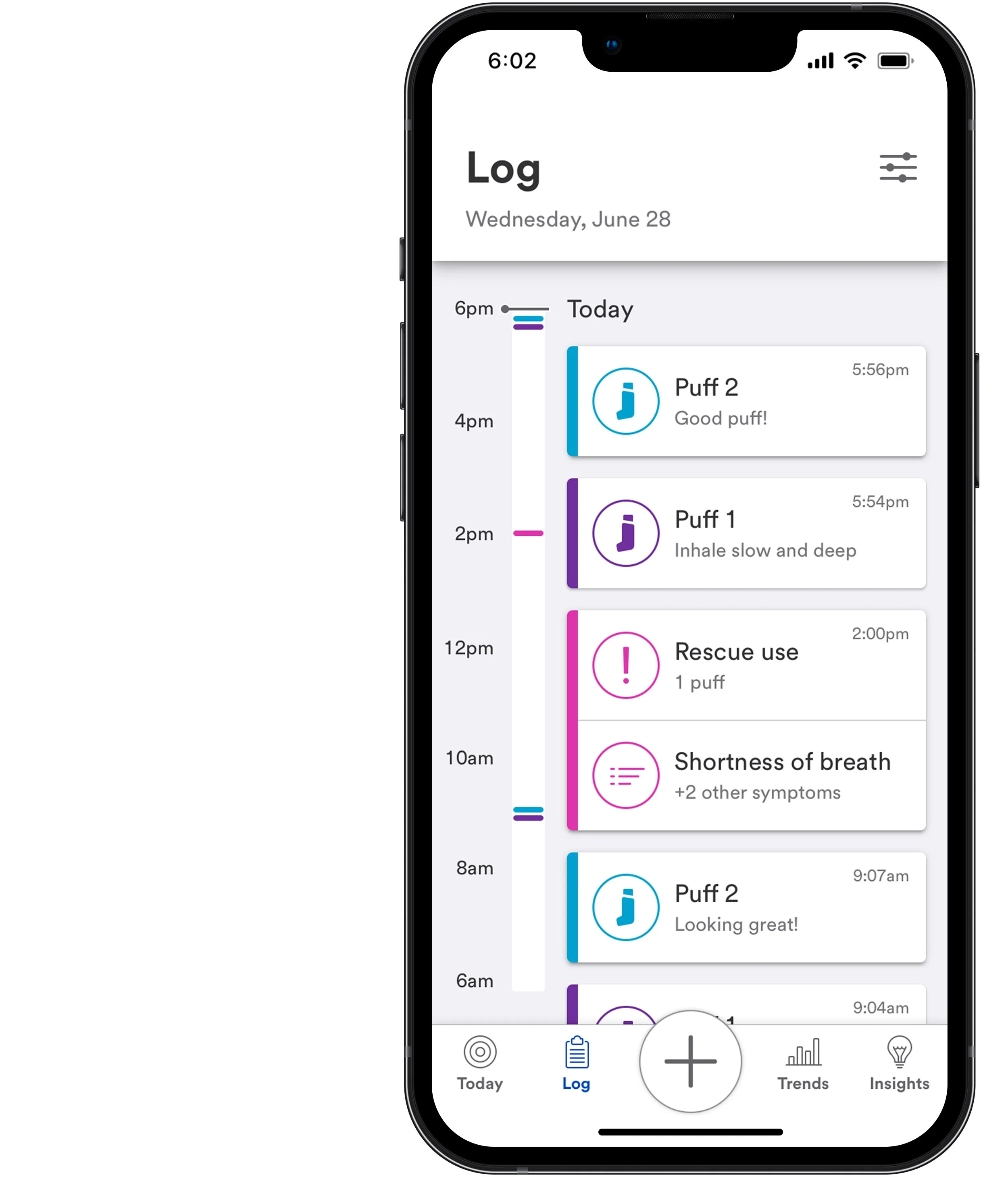
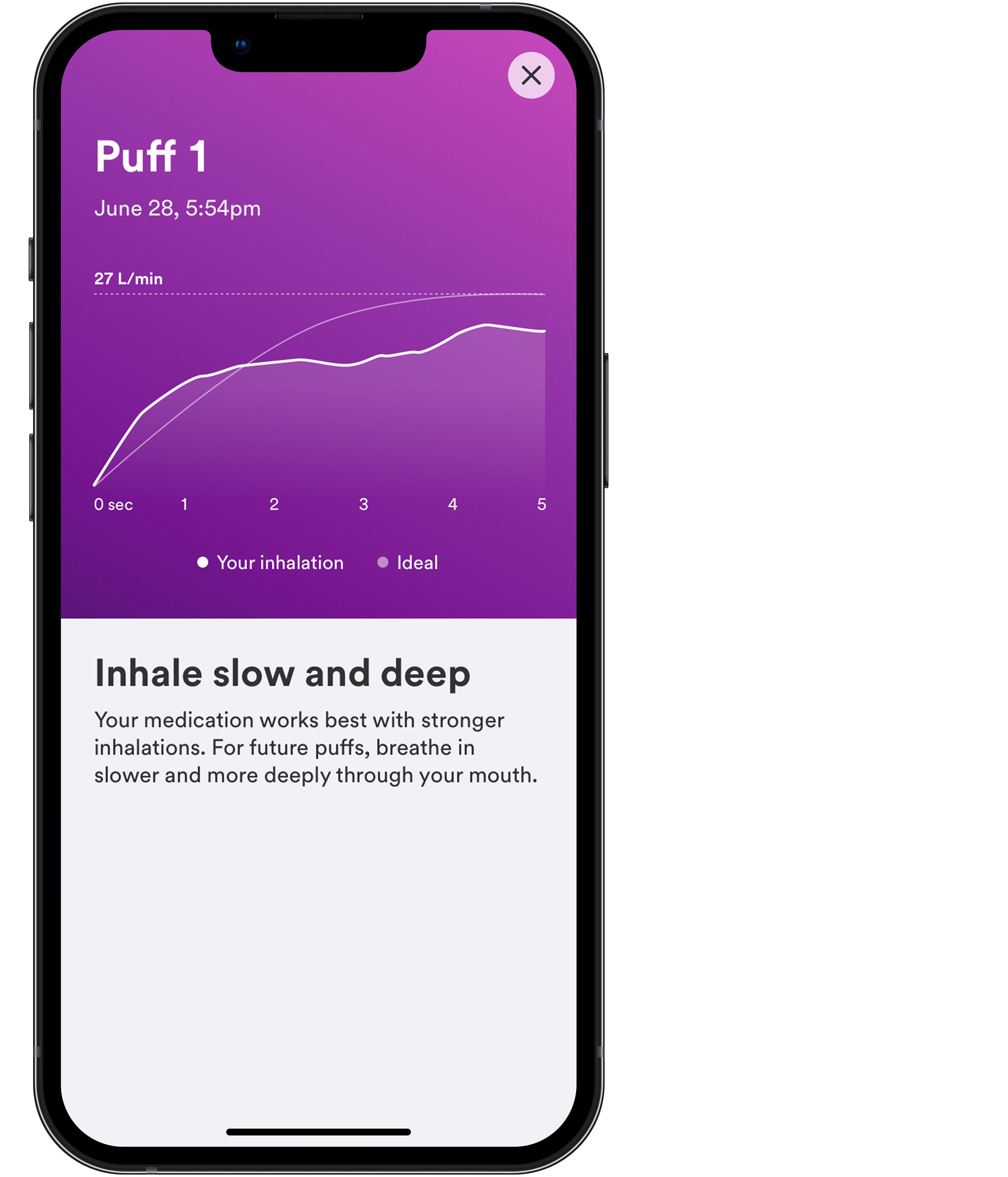
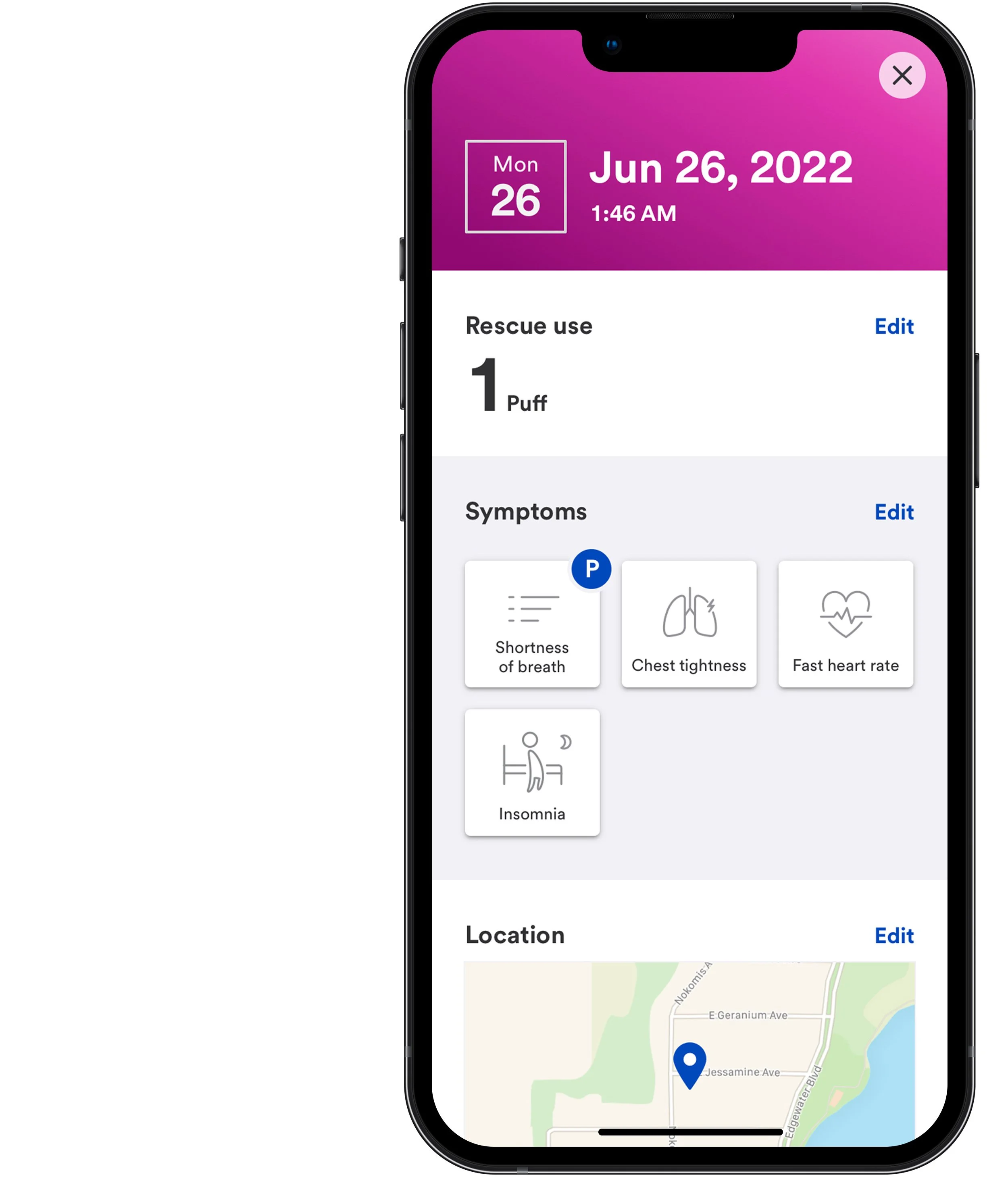
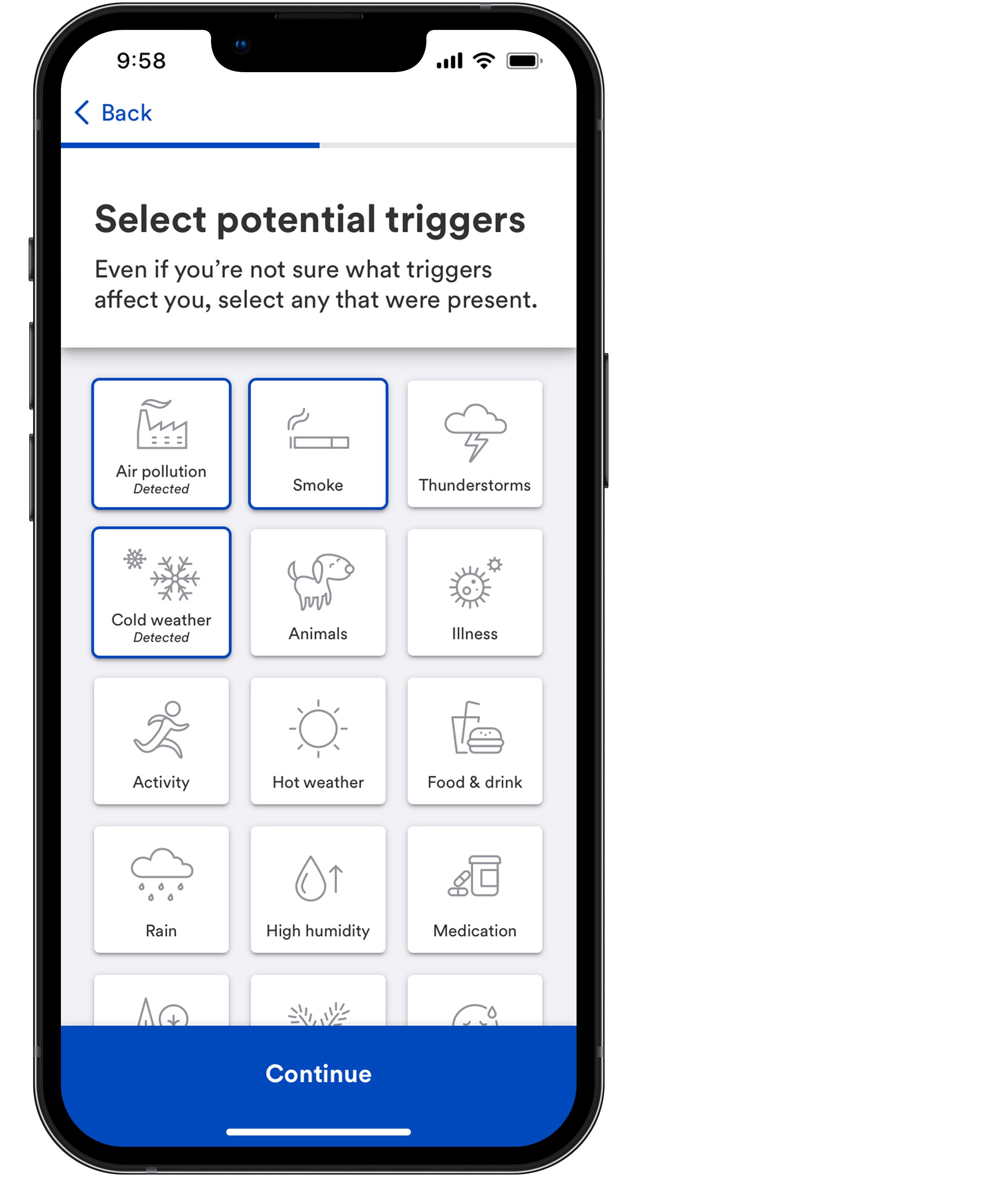
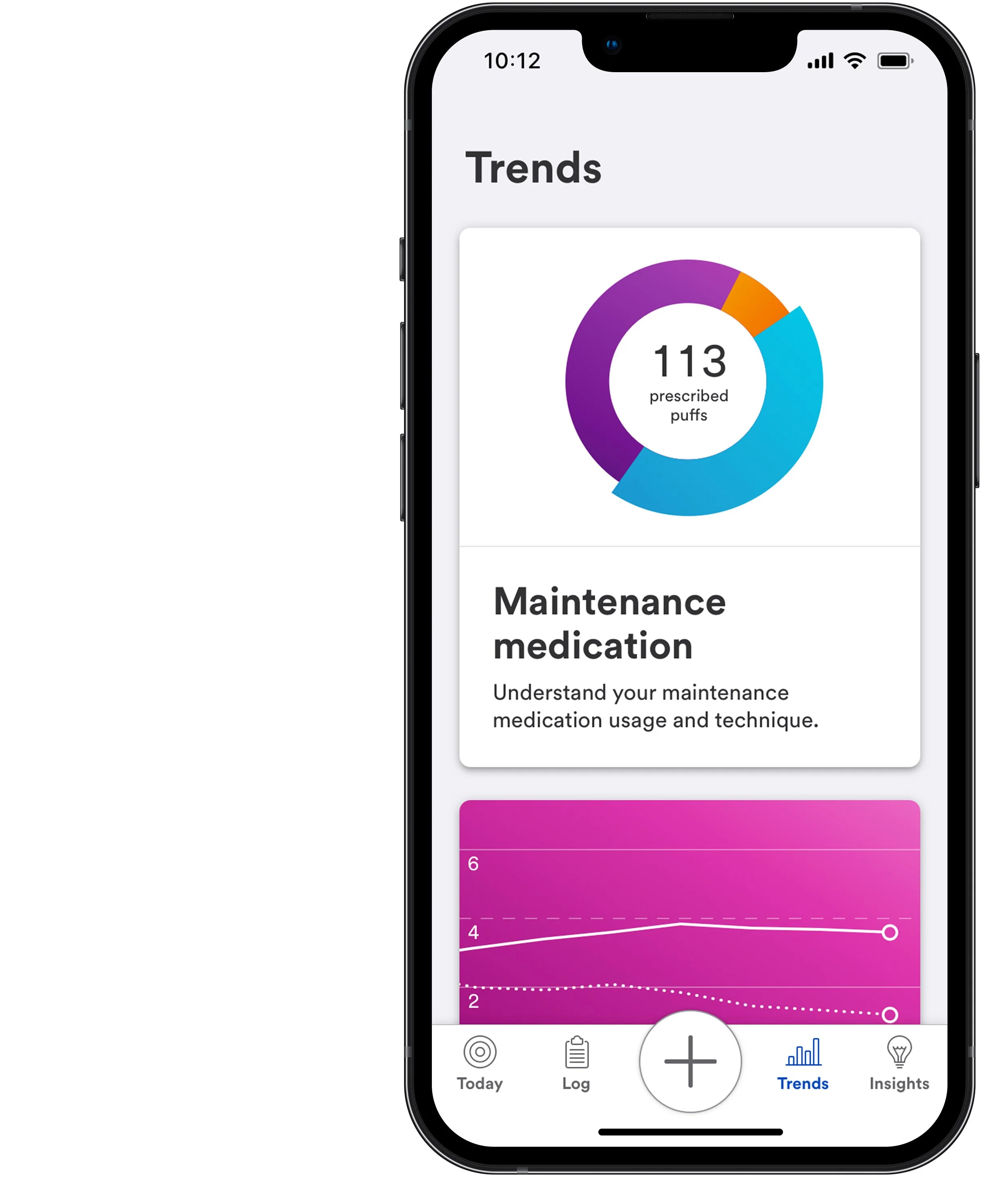
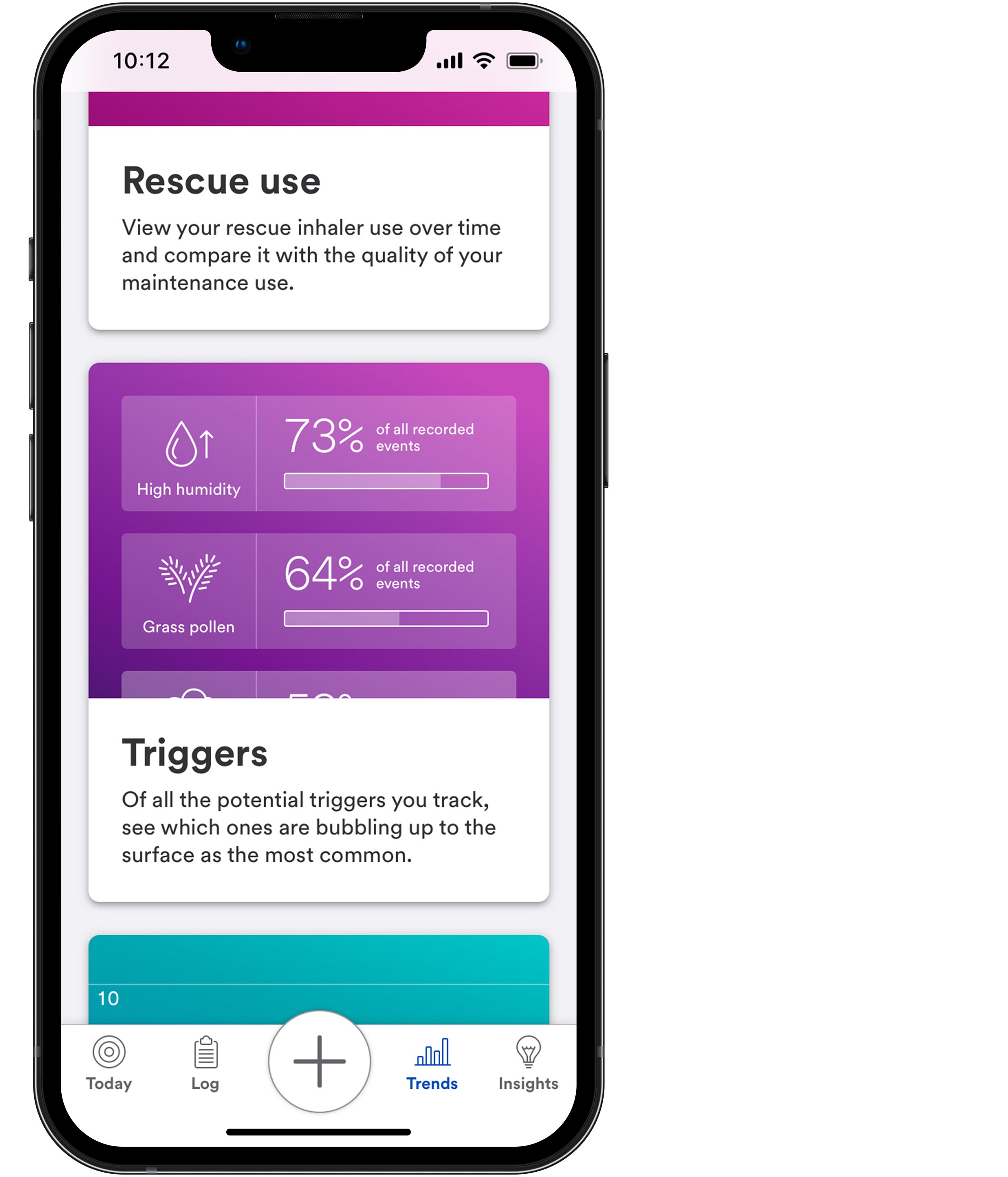
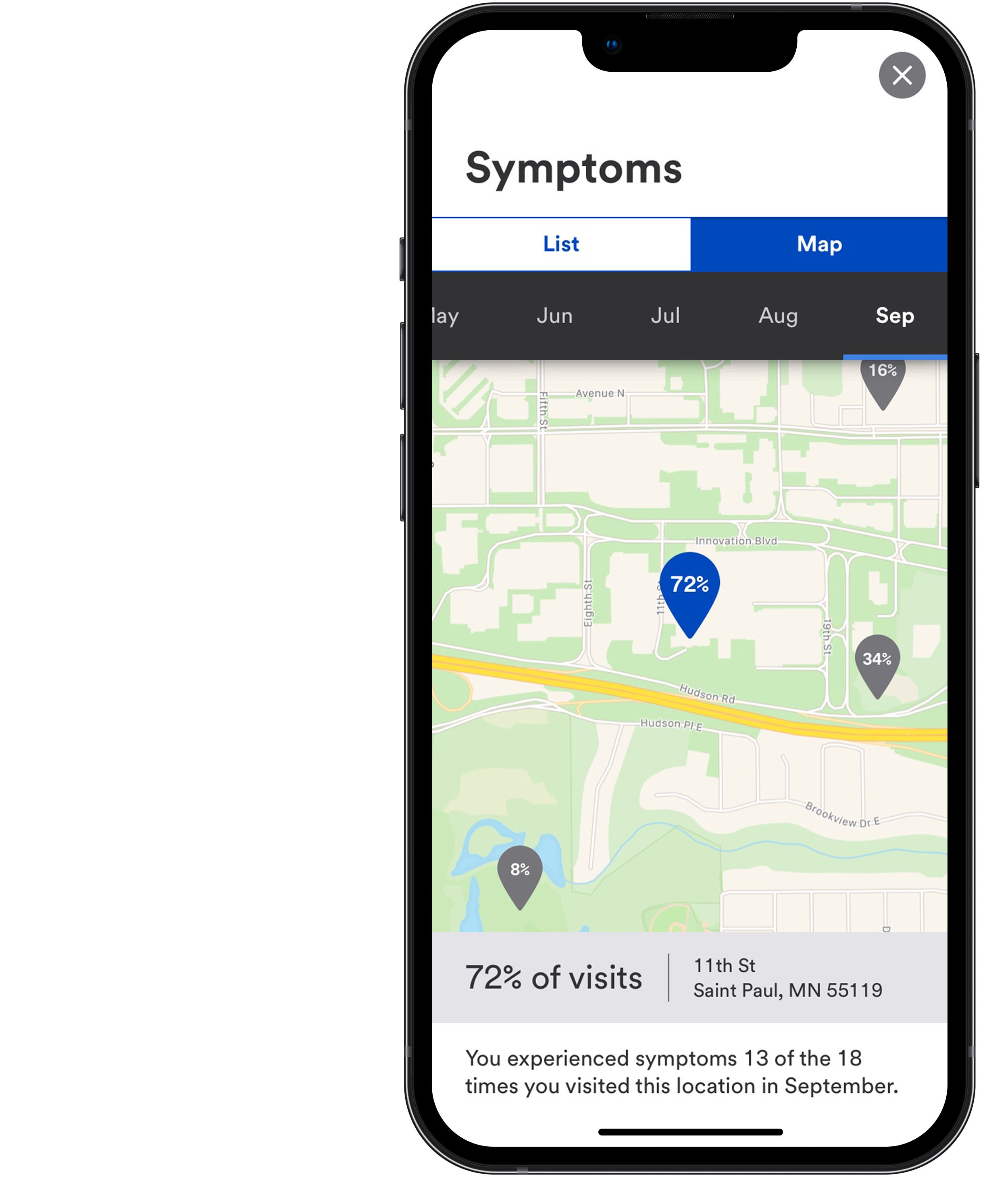

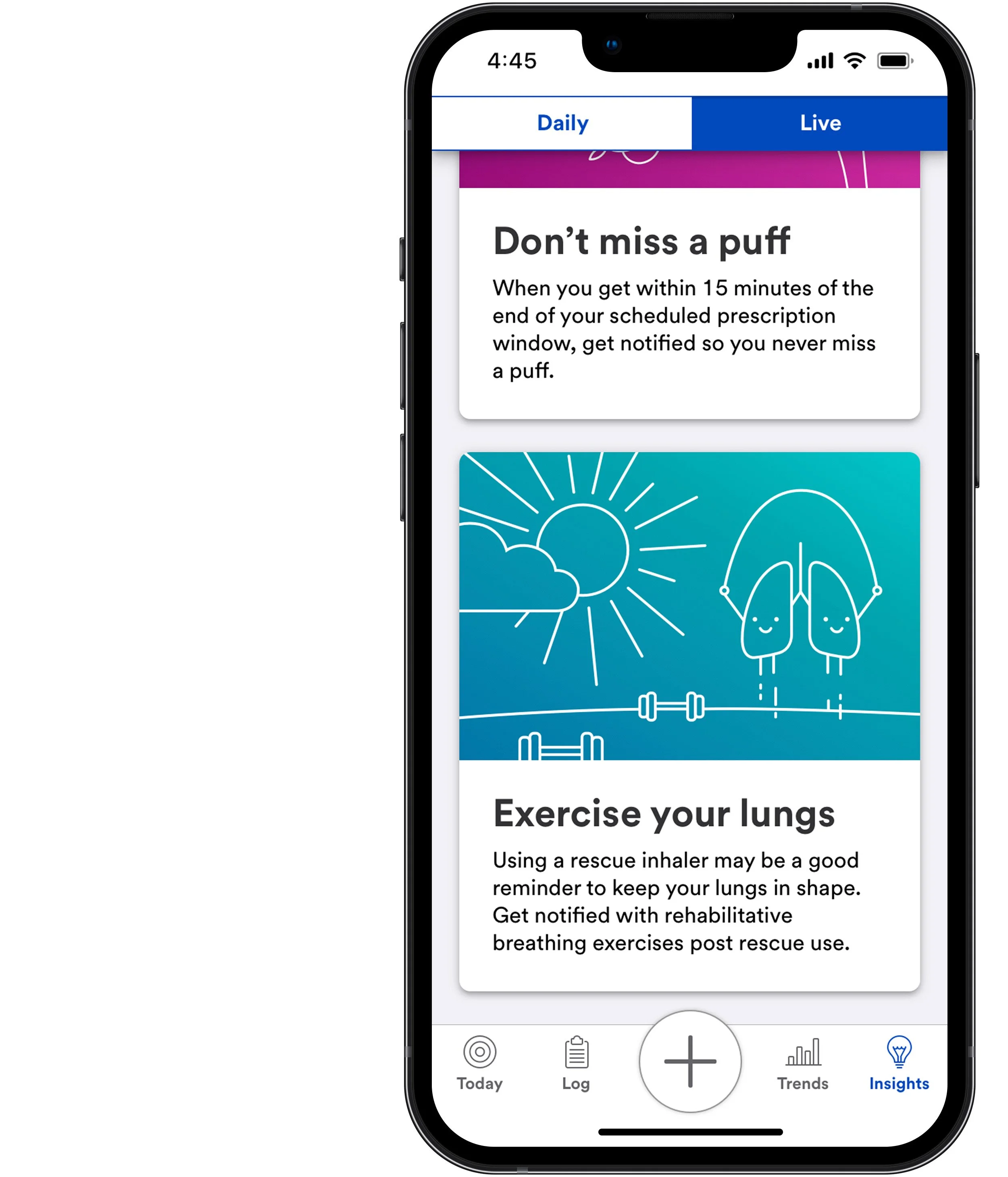
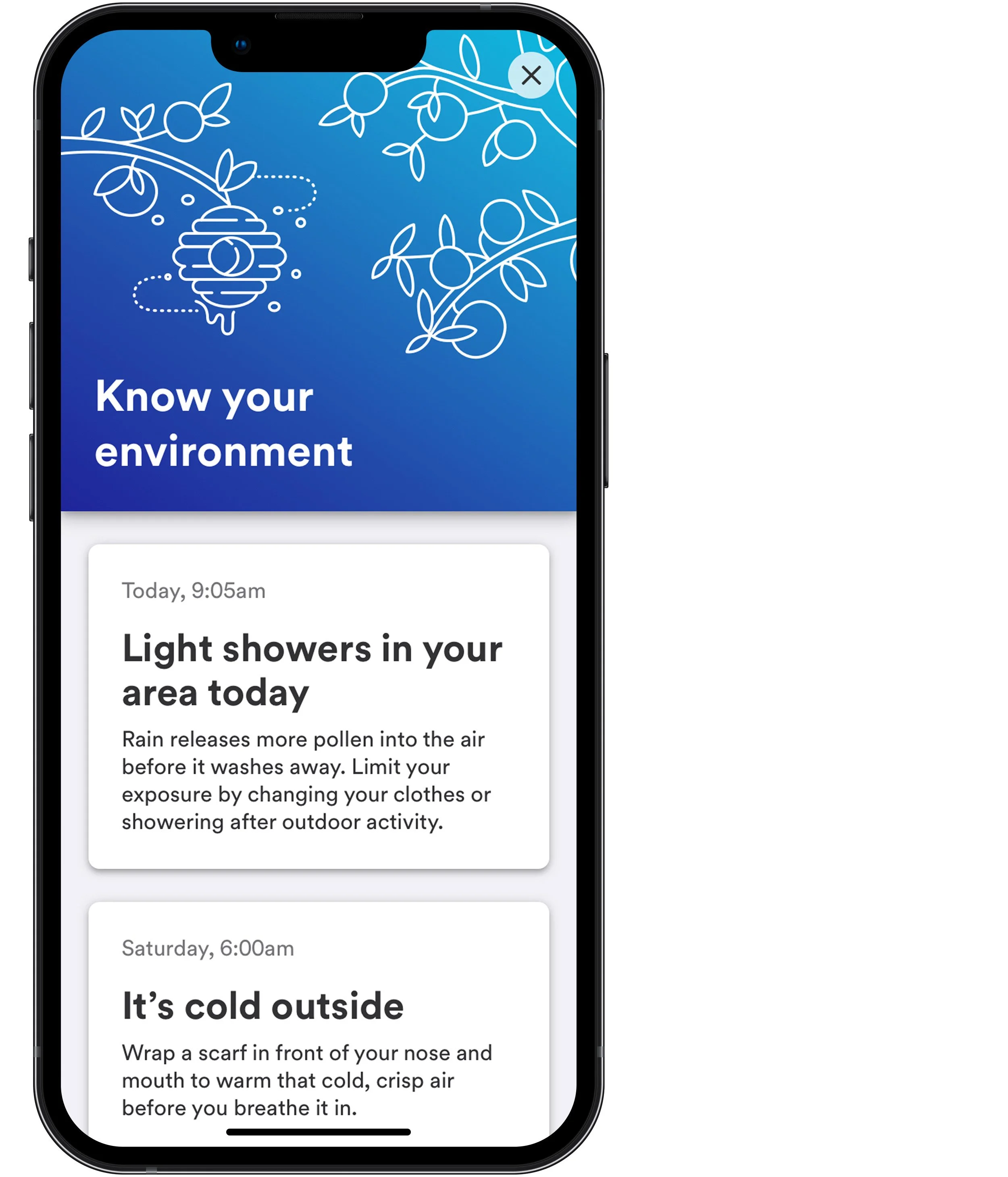
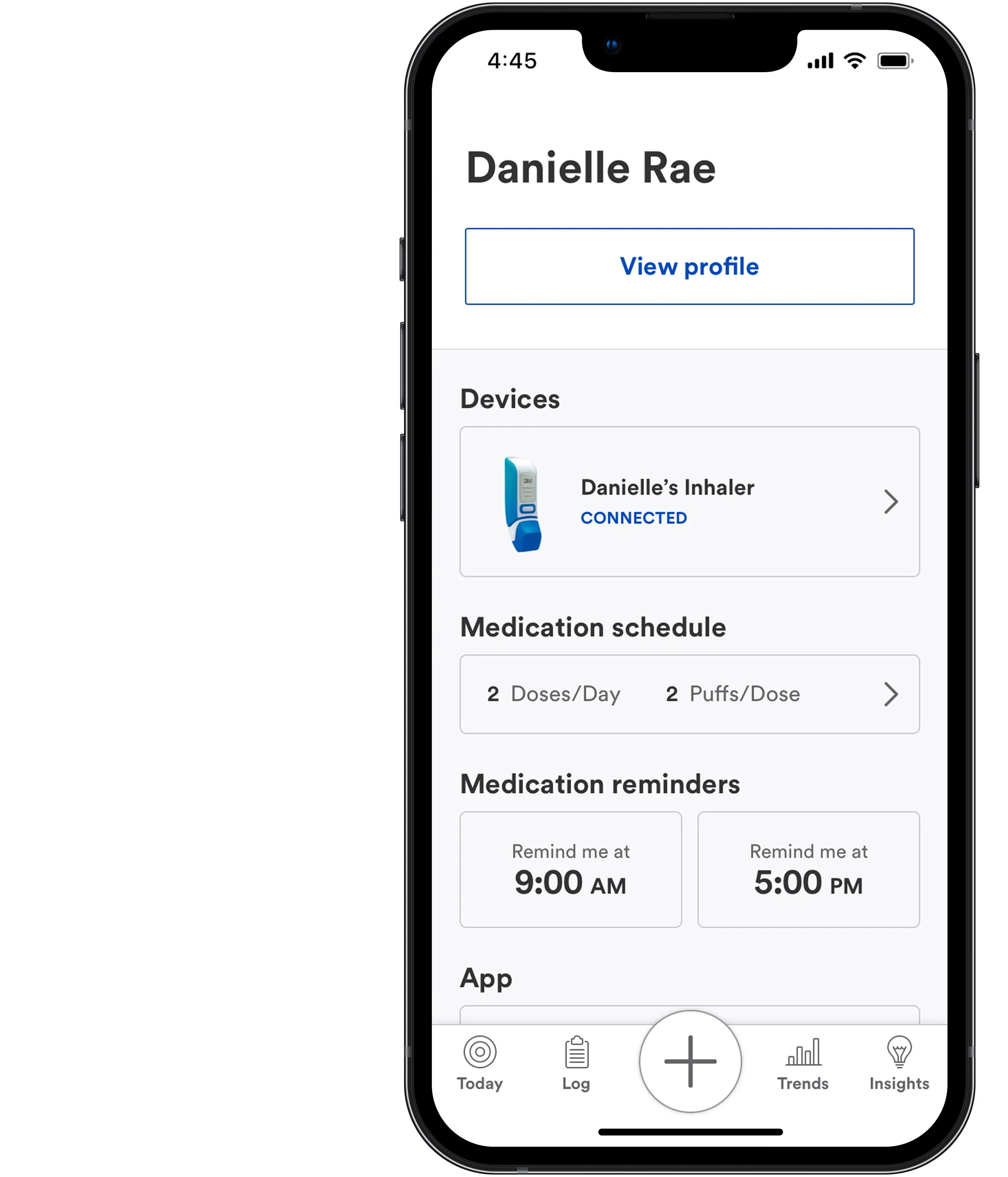
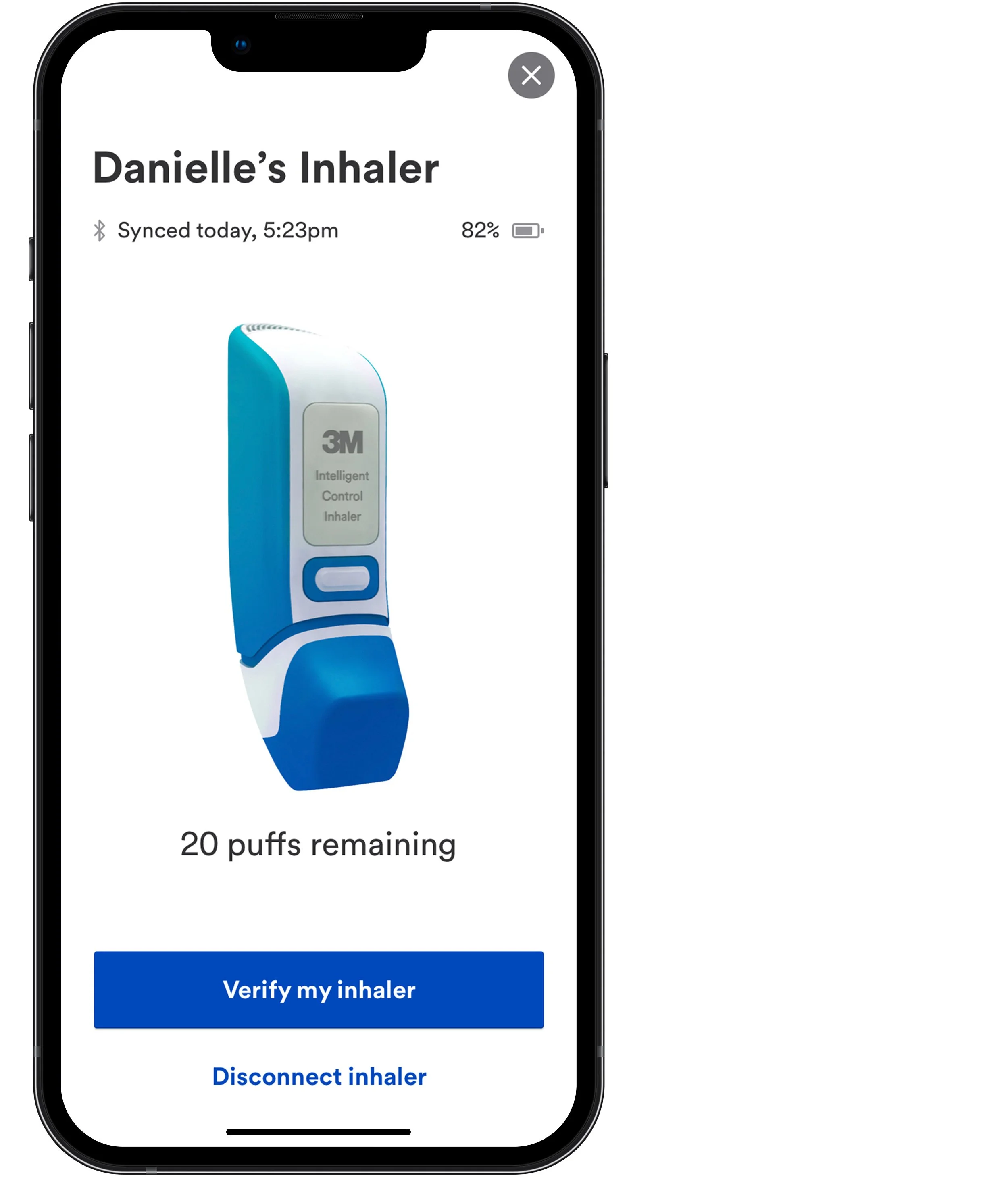
Sources
Schluger, Neil W, and Ram Koppaka. “Lung disease in a global context. A call for public health action.” Annals of the American Thoracic Society vol. 11,3 (2014): 407-16. doi:10.1513/AnnalsATS.201312-420PS
Barnett S and Numagambetov T. “Costs of asthma in the United States: 2002-2007.” JACI. 2011. Jan:127(1):145-152. doi:10.1016/j.jaci.2010.10.020
Guarascio, Anthony J et al. “The clinical and economic burden of chronic obstructive pulmonary disease in the USA.” ClinicoEconomics and outcomes research : CEOR vol. 5 235-45. 17 Jun. 2013, doi:10.2147/CEOR.S34321
DiMatteo MR. “Variations in patients’ adherence to medical recommendations: A quantitative review of 50 years of research.” Med Care 2004;42(3):200-209.
Laube, B L et al. “What the pulmonary specialist should know about the new inhalation therapies.” The European respiratory journal vol. 37,6 (2011): 1308-31. doi:10.1183/09031936.00166410
Lindsay, John T, and Liam G Heaney. “Nonadherence in difficult asthma - facts, myths, and a time to act.” Patient preference and adherence vol. 7 329-36. 19 Apr. 2013, doi:10.2147/PPA.S38208
Lavorini, Federico et al. “Effect of incorrect use of dry powder inhalers on management of patients with asthma and COPD.” Respiratory medicine vol. 102,4 (2008): 593-604. doi:10.1016/j.rmed.2007.11.003
Biswas, Rajoshi et al. “Factors Determining In Vitro Lung Deposition of Albuterol Aerosol Delivered by Ventolin Metered-Dose Inhaler.” Journal of aerosol medicine and pulmonary drug delivery vol. 30,4 (2017): 256-266. doi:10.1089/jamp.2015.1278